Tapping into the future.
While I worked with product invention lab Handmade, we routinely ran internal R&D sprints with small teams of digital/physical product designers. One time, we used voice and image recognition and smart sensors to turn a dumb tap into a smart(er) one.
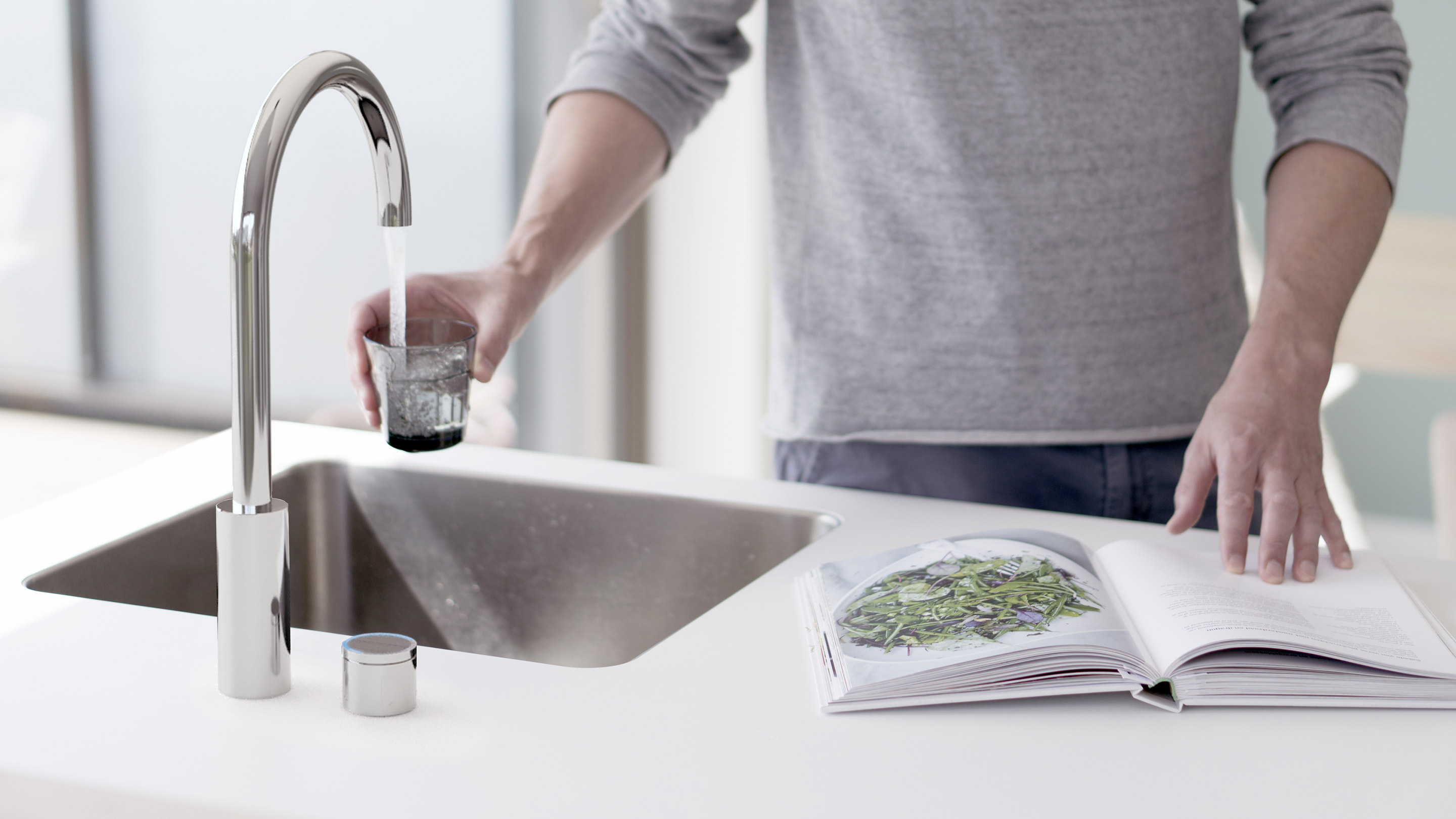
As a product designer, one of our most valuable assets is a critical look at the world around us. To notice the little friction points and inefficiencies that everyone else takes for granted, and design better solutions.
And this matters, because we are surrounded by mediocreness. Many things we use in our daily lives haven’t been improved for decades. They are neither delightful, nor particularly problematic. We simply fail to see their shortcomings because they have have always been this way.
A closer look at taps
Taps are a great example. They have no obvious pain points. It’s only when you look closer you start noticing little points of friction:
- you have to use two hands
- you have to wait while it's running
- you need a measuring cup to get exact amounts
- you are often left with splashes and puddles around the sink
(Oh wait—here is a pain point: burning your hands because someone before you used hot water and there is no way you could tell.)
Design for intent
In this case, I was particularly intrigued by using cues in our natural behaviour to derive intent. For example, a natural way to convey “Okay this is enough water, thanks!” is to move the glass away from the tap.
What if... a tap would just know what we want, by guesstimating our intent from our natural behaviour?
To quickly get a feel for how a “context-aware” tap could work, I explored the key interactions, flows and behaviours for major use cases in a low-fidelity format that encouraged quick iterations.
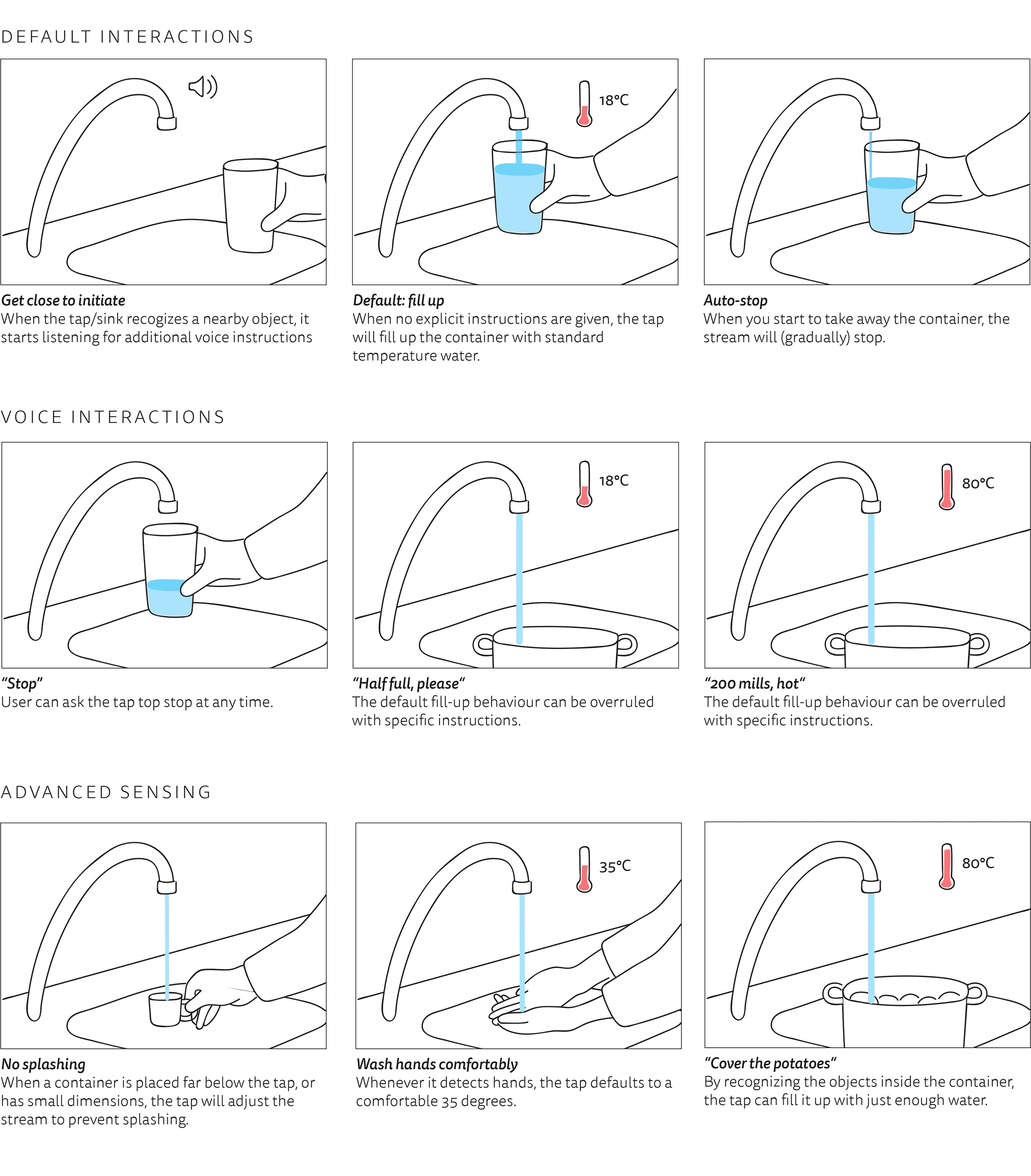
Towards a prototype
Since these improvements are difficult to prove in Illustrator, we went on to build a fully-functioning prototype to validate our assumptions.
As our design ideas relied heavily on object recognition to distinguish espresso cups from cast-iron pans, one of our technologists built a relatively simple image-classification model in Tensorflow.
Pairing our object recognition model with a wide-angle camera, depth and water flow sensors and a bit of old-school plumbing, our team put together a working prototype in a matter of days.
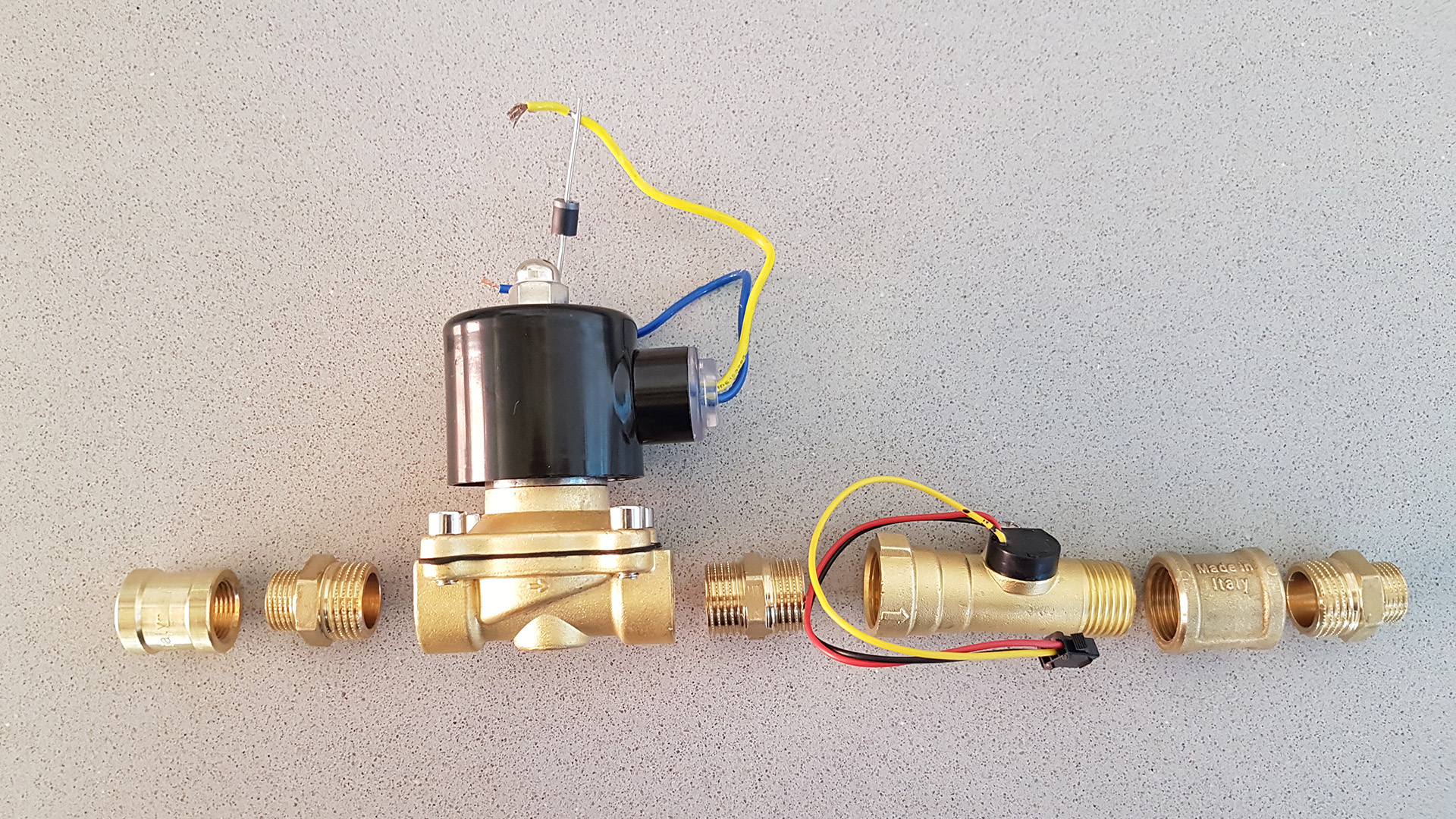
We also fitted our prototype with simple LED-ring as a feedback mechanism to indicate things like the current water temperature, or the amount of upcoming water when filling a container.
Despite occasional software and hardware hickups, the actual user experience when the prototype worked as intented proved nothing short of magical.
Now what?
Aside from improving our design, prototyping and engineering skills, this project was succesful in that it proved to us that—using relatively simple, affordable technology—it is indeed possible to fundamentally improve an otherwise unremarkable, everyday household appliance.